Use cases for an advanced approach to monitoring chemical plants
How single-sensor anomaly detection works in intelligent plant monitoring systems. We look at the real-world benefits from chemical plants for use cases such as compressor and valve monitoring, and the potential for plant teams to avoid downtime incidents.
Techniques and use cases
The first article in this series looked at the benefits of using data-driven systems for 24/7 monitoring of chemical plant environments and proactive alerting.
It highlighted how one such system, the Navigance Plant Monitor, helps teams running those plants to identify patterns and behaviours of concern in their data early. Equipped with early warnings about anomalies and deviations, they can take proactive, remedial action to avoid unplanned downtime.
Now let’s look at some of the methods used to realize these benefits and use cases that illustrate how they work in practice. We’ll cover:
- How anomaly detection works
- Single sensor monitoring and anomy detection
- A compressor monitoring use case
- A valve monitoring scenario
The next article in this series will focus on the benefits of multi-sensor monitoring in chemical processes.
Plant monitoring approaches
The Navigance Plant Monitor takes several approaches to identifying anomalies and deviations that may pose a concern. This range of techniques ensures a broad scope for detection and analysis, so we can tailor deployments to each plant’s specific monitoring needs.
To start with, let’s focus on one – sensor anomaly detection – and the benefits producers have experienced using this approach in different scenarios and for different applications. Some examples origin from benefit assessments Navigance has performed for customers to quantify the gains that can be made detection time for events such as equipment failure.
How anomaly detection works
The Navigance Plant Monitor uses a variety of underlying technologies to enable intelligent plant monitoring. One concept is pattern detection, which involves detecting repetitive and similar patterns, as well as changes in their frequency or sudden changes in their distribution.
Figure 1 below shows an anomaly detection method in which time-series data is screened for similar, and time intervals that show unique and, as such, anomalous characteristics are isolated. An alternative approach to search for patterns that in themselves appear anomalous.
Using this method, single or multiple sensors can be monitored for anomalies. Whole sensor groups can also be monitored for consistency.
Figure 1: Time series anomaly detection finds ‘normal’ patterns, then isolates anomalies
Single sensor monitoring and anomaly detection
In single sensor anomaly detection, Navigance algorithms continuously monitor the time-series data from sensor signals for one or multiple search patterns that are known to commonly indicate critical process or equipment conditions.
Figure 2 illustrates the wide variety of possible search patterns and methods Navigance uses to detect anomalies. For every anomaly that is confirmed, the data gets enriched with annotations linked to a specific time or timeframe.
Figure 2: Single-sensor anomaly detection searches for known anomalous patterns.
Example use case: compressor monitoring
One real-world example of how single sensor anomaly detection can be applied is in monitoring the pressure signals from compressors. In any chemical plant, compressors are among the most critical equipment. If they fail, the downtime caused often results in a total plant shutdown and significant loss of production.
Figure 3 shows the example of a compressor failure in a methanol plant. Ordinarily, the plant would face unplanned downtime while compressor pistons and rider rings were replaced. However, when Navigance applies single sensor anomaly detection algorithms to the plant’s data retrospectively, it shows the outcome could be very different with intelligent plant monitoring in place.
Figure 3: Single sensor detection monitors a compressor pressure signal. A warning and alert are triggered weeks before the need for major repair, which would otherwise require an unplanned shutdown (see dashed red line).
The graphics shows how our Plant Monitor identifies a long-term pattern that reveals a compressor unit is malfunctioning. Signal degradation is occurring over several months, making it practically invisible to personnel checking on a daily or weekly basis. With such patterns rarely monitored at all, the likelihood of failure and downtime would ordinarily be high.
Here, though, specific Navigance algorithms are able to identify the anomalous pattern over a longer timescale and highlight continuous increases in oscillation from the compressor’s pressure signals (see the funnel highlighted red) before the unit failed.
The Plant Monitor generates an initial warning two months before the failure, and a second, more urgent alert two weeks before the event. Armed with this information, the plant team would be able to proactively arrange spare parts and schedule planned maintenance, intervening early to avoids more severe damage, reduce repair time, and minimize lost production.
Another example: valve monitoring
Changes in signal patterns can also provide early warning signs of damage to equipment, such as valves. The example in figure 4 shows the output of a controller unit for the valve position, where damage to a valve that occurred in September went unnoticed until mid-December, when an unscheduled major valve repair had to be carried out.
Figure 4: Illustrates the changes in a signal’s pattern from its normal state (P1) to anomalous (P2). Protective alerting to such changes help plant teams identify and resolve equipment damage to minimize its impact.
By again applying our pattern detection models to this data set retrospectively, Navigance is able to show that the first unique and anomalous patterns could be identified a full two months before the unscheduled repair. A critical signal dynamic is also detected one month before the failure, triggering an alert based on its severity.
Again, these early warnings enable the plant team to investigate this further, schedule maintenance, avoid extended damage periods and reduce repair demands.
Ready to find out more?
These are just two example use cases for single sensor monitoring, which illustrate common failures and malfunctions in chemical processes that the Navigance Plant Monitor can help detect and mitigate against.
In the next article, we dive further into the opportunities for applying algorithms to more complex, multi-sensor monitoring scenarios.
If you’re already keen to know more about the potential of plant monitoring or discuss specific issues that cause downtimes in your plant, contact our Navigance experts.
Read the other articles in this series:
Use cases: Multi-sensor anomaly detection
Don't miss out on new articles.
Explore further:
How to avoid the costs of unplanned downtime
$20 billion. That’s how much unplanned downtime is costing the chemical industry each year by some estimates. Yet producers continue to tackle issues reactively instead of spotting and fixing them early, before they become problems. The key to doing so lies in your data – and the Navigance Plant Monitor helps you turn it.
Whitepaper: Advance with intelligence
AI-enabled data analysis unlocks hidden process optimization potential, effortlessly and without tying up your resources. Our free white paper reveals where to start.
Whether you’re responsible for operating a chemical plant, part of the engineering team that keeps it up and running, or you oversee the commercial aspects of its wider business, one thing is increasingly certain: you need to digitalize sooner or later.
Read more
Optimizing chemical production using data-driven, AI-enabled hybrid modeling
Smart use of your plant’s data can take process efficiency to a higher level. Hybrid models driven by artificial intelligence deliver the best results for the least effort. And you don’t need a data science degree to enjoy them.
Read more
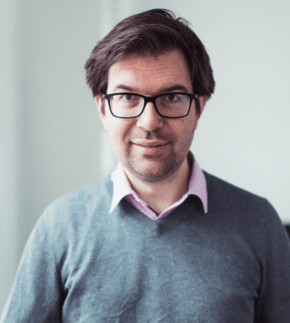