Optimizing chemical production using data-driven hybrid modeling
Smart use of your plant’s data can take process efficiency to a higher level. Hybrid models driven by artificial intelligence deliver the best results for the least effort. And you don’t need a data science degree to enjoy them.
Data is everywhere these days – and the volumes of it are growing exponentially all the time.
We’re used to smart uses of it in our everyday lives. Like Amazon and Netflix using our past behavior to tempt us with new products or shows, even making them more appealing by tailoring how they look based on carefully constructed, data-driven profiles of our tastes. Much of that heavy lifting is done by artificial intelligence (AI).
In chemical production, process control systems have been collecting data measurements during continuous or batch manufacturing for decades.
This information, and other data in the operation, could be key to unlocking untapped potential or driving industrial innovations. But collecting it is one thing. Using it – and using it effectively – is quite another.
Beyond some limited, usually irregular applications, such as helping operators troubleshoot, or facilities develop KPIs, use of such measurements remains sparse. Often the data is simply stored by historians.
In fact, only a small amount of chemical process data is being harnessed effectively to enable timelier, more informed decision-making. And that’s because, as in so many other sectors, most producers are data rich but information poor.
Finding meaning in the numbers
Let’s assume for a minute that your plant already collects lots of data from across its production process – a substantial task in itself we’ll discuss elsewhere later.
The key challenge then is usually: “What on earth do we actually do with all of that data?” Or, more precisely, how do you handle and structure vast volumes of it? How do you siphon out what’s irrelevant and focus on what’s essential? Extracting meaningful insights you can act on with confidence to optimize operations.
True data-driven decision-making would not only avoid unnecessary performance and efficiency losses, but also help find and unleash previously untapped potential.
Advanced analytics make it possible, enabling you to make and execute good decisions faster and more frequently, and – using the most robust yet flexible approaches – optimize continuously.
Advanced analytics for continuous processes
Many analytics solutions focus on batch production, but there are a growing number of advanced analytics options – and quite different approaches – for continuous chemical processes too. It’s important to understand the differences, and which can offer you the greatest gains for the least effort and investment.
Do it yourself: self-service analytics
Standard work tools such as Microsoft® Excel spreadsheets are a handy way to get a first glimpse of your data, but little more. Few production sites can spare the time and resource needed to try.
Those that do likely check at irregular intervals and optimize mainly individual aspects of their process. Even then, they struggle to find meaning in, and draw conclusions from, the numbers because these tools quickly reach their limit when more advanced analytics are needed.
Recently, more sophisticated self-service tools have brought a new level of scrutiny to continuous process data, including more holistic, frequent and even continuous checks. Don’t underestimate the investment in people and training they need, though. In-house experts must dedicate both time and deep process and data science know-how to harness them effectively.
In short, the more manual and self-service approaches to data analysis leave users to perform limited, ad hoc analysis themselves and draw their own conclusions on which to act. Other approaches can take that weight off their shoulders.
Laying the foundations for intelligent analysis
New tools and technologies enable much more precise and effective analysis, but they take fresh thinking too – like the courage to go beyond traditional ways of working.
You may have heard of or already be using ‘first principle’ models for process optimization. These well-established techniques swap the qualitative guesswork common to plant design with quantitative calculations based on physico-chemical relationships to determine plant properties.
It’s not easy, and certainly not perfect. Ensuring parameters will apply in real-world situations can be expensive and time intensive. Even then, the many complex relationships stacked on top of each other in a typical plant can still leave uncertainty and render calculations inaccurate.
As such, first principle models rarely present a truly ‘real-world’ view of operations, or the parameters they do set can be all over the place. They lack the flexibility needed to adapt to occasional adjustments to the plant’s setup and constant changes in how it operates – such as those due to, for instance, seasonal effects, catalyst deactivation* or equipment fouling.
Partnering with intelligence
Adding artificial intelligence to the mix creates opportunities to interrogate plant data holistically, present findings and recommended actions, and inform smarter decisions.
AI is not the answer alone, as it’s of no real practical use without proven domain knowledge to interpret and verify it – especially in a space as complex as chemical manufacturing. So you need to think of artificial intelligence as a partner in process optimization.
Hybrid models of your plant offer a way to start doing so. They combine first principle techniques with AI to create a foundation for optimization that’s driven by real-world, real-time data, highly flexible to change. And they pave the way to full-service analytics.
Full-service analytics from Navigance
The Navigance approach enhances established first principle models by adding machine learning. The result is AI-enhanced hybrid models that are informed by real-time plant data and offer unprecedented adaptability.
Unknowns are carefully built in, using arbitrary functions to create a good fit for all observed data and capture all relevant effects. Any insights generated are of a high predictive quality, and operators can act on them with confidence to optimize operations.
What’s the catch? Well, such hybrid modelling still requires detailed knowledge of chemical processes and data science. That resource demand isn’t one you must bear, however. Navigance combines not just the tools needed to make sense and better use of your data, but also that most scarce of resources: people.
With years of combined experience, our experts will implement a hybrid solution that suits your plant’s setup and parameters, learns patterns and spots potential inefficiencies in your process, and delivers reliable recommendations to keep it running at its best.
It doesn’t end there, though. As a truly full-service analytics solution, Navigance provides ongoing support from our team of process and catalyst experts, on tap when needed. Keeping your team free to concentrate on running your operation, and helping to continuously optimize it today and tomorrow, even as conditions change.
___________
* Some companies have invested substantial time and energy in developing sophisticated first principle models yet acknowledge using them for continuous and dynamic process optimization is far too complex. In discussions with one methanol producer we came to know that, despite publishing extensive results from its first principle model investigations, the producer had not achieved further improvement over the last 10 years because describing catalyst deactivation in an industrial plant operation is too complex.
Explore further:
Chemical process models – from first principle to hybrid models
In this article, we explore the role, common uses and limitations of the first principle approach to chemical process modelling. And we contrast this with the advantages and disadvantages of using empirical or statistical models.
We then look at how taking the first steps into new, intelligent 'hybrid' models can deliver the best of both approaches and more than the sum of their parts.
Read More
This might interest you too.
> Bain: Creating value through advanced analytics
> Bain: How to choose among three forecasting models
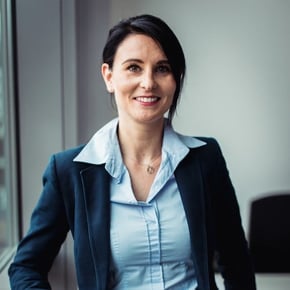